2D&3D Vision Analytics
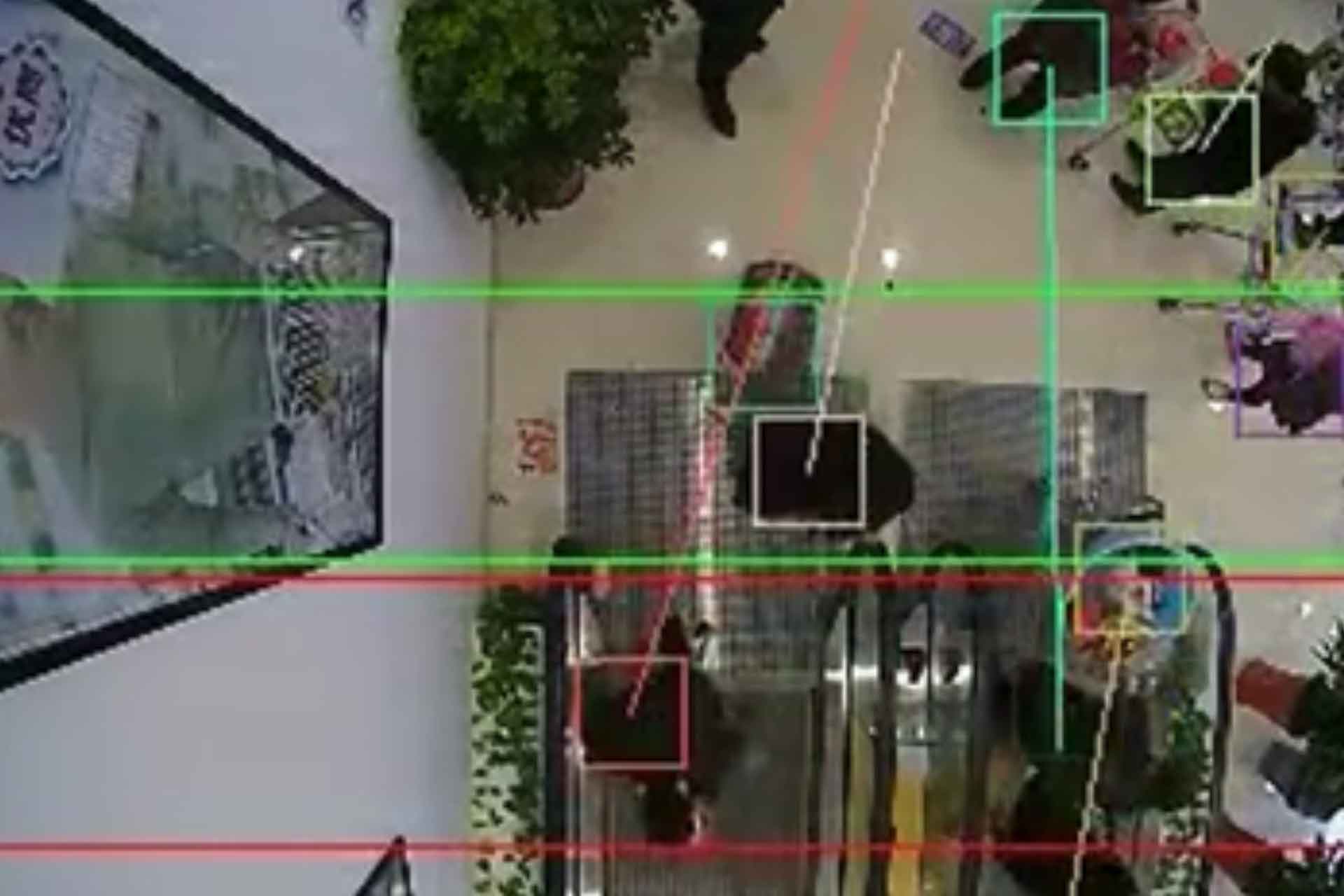
How Does TD Intelligent Sensor Work?
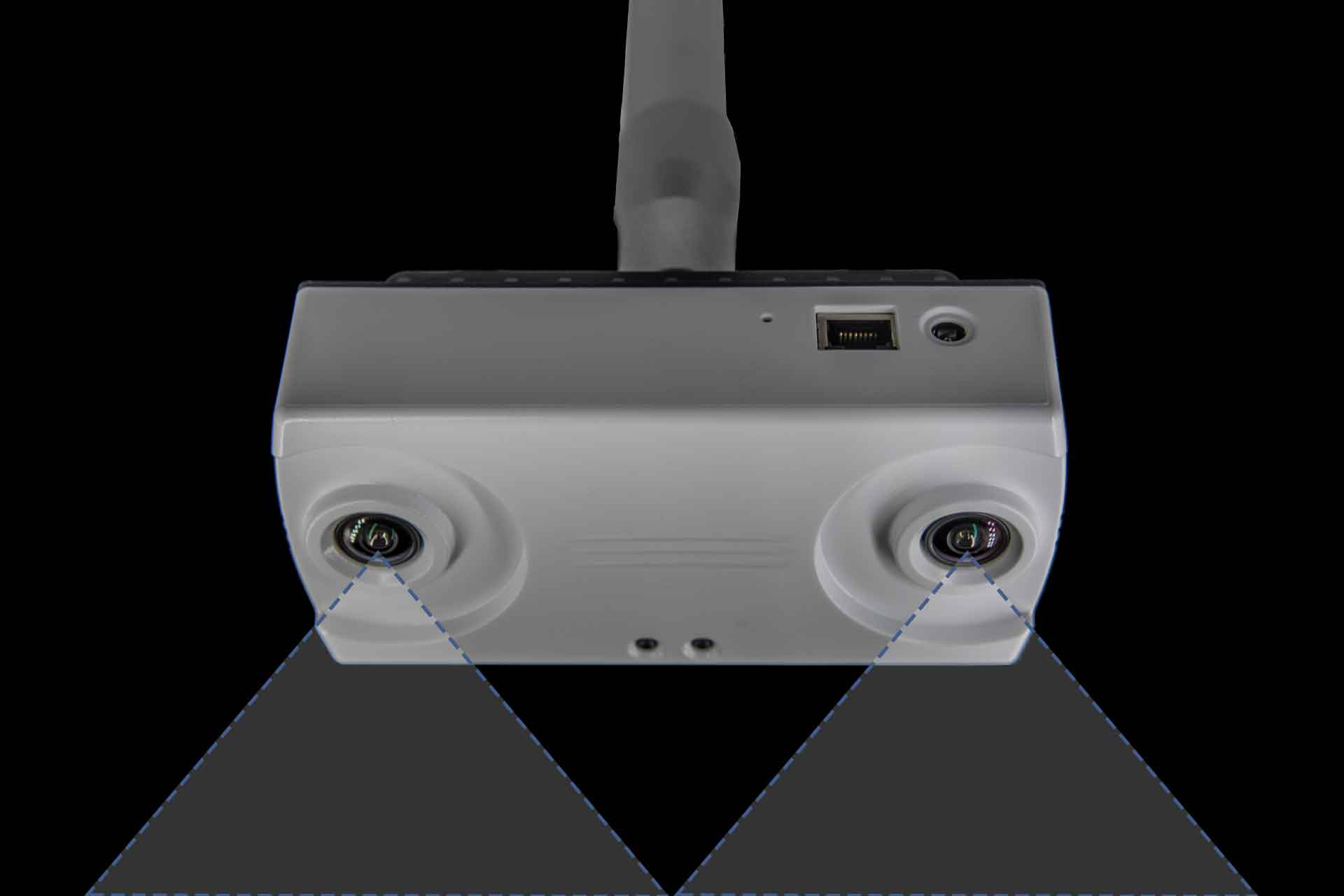
3D Stereo Vision Intelligent Sensor
“DeepCount” Based on Deep Learning
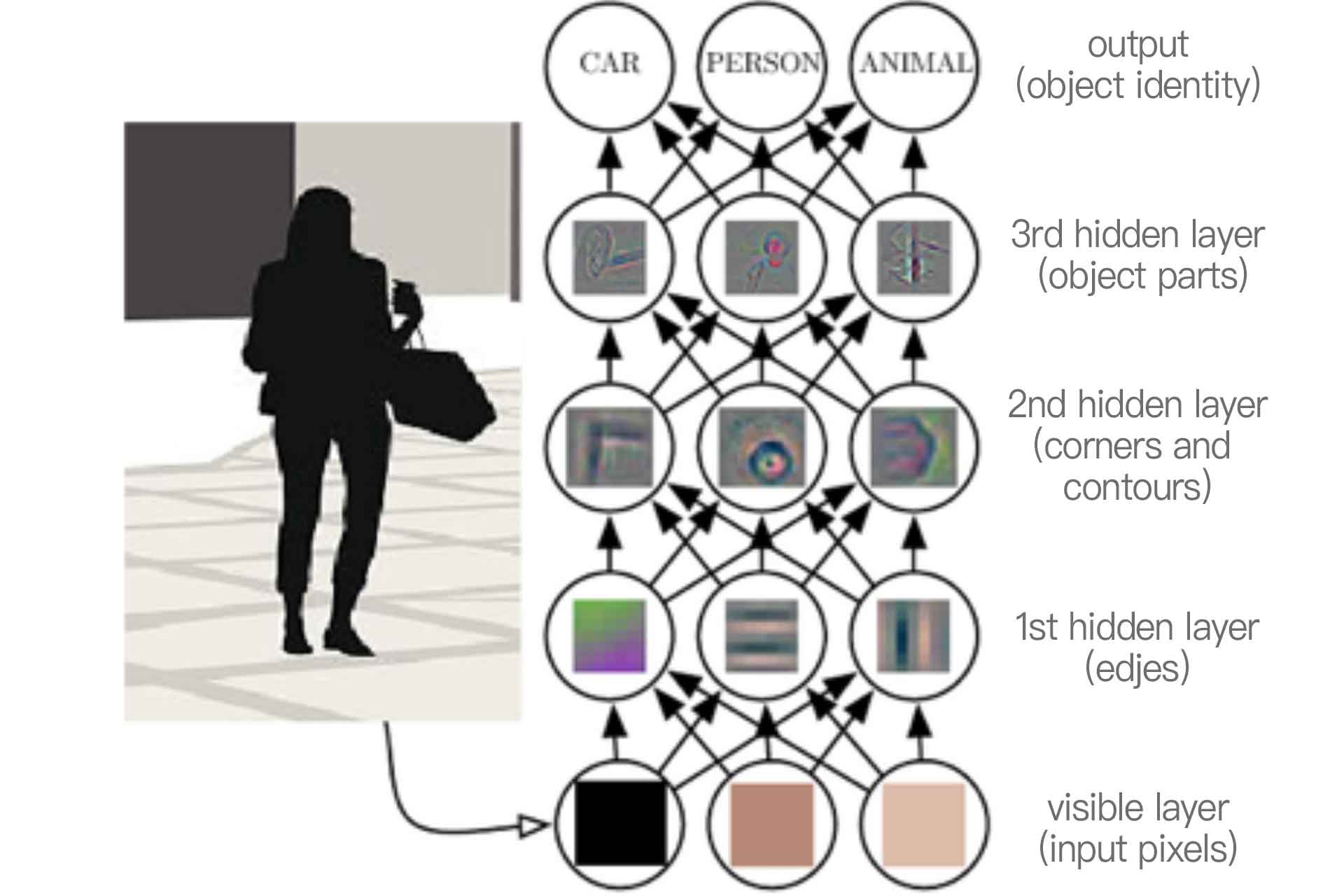
Illustration of Deep Learning
How Does Deep Learning Work?
Limitations of Deep Learning Applied For People Counting
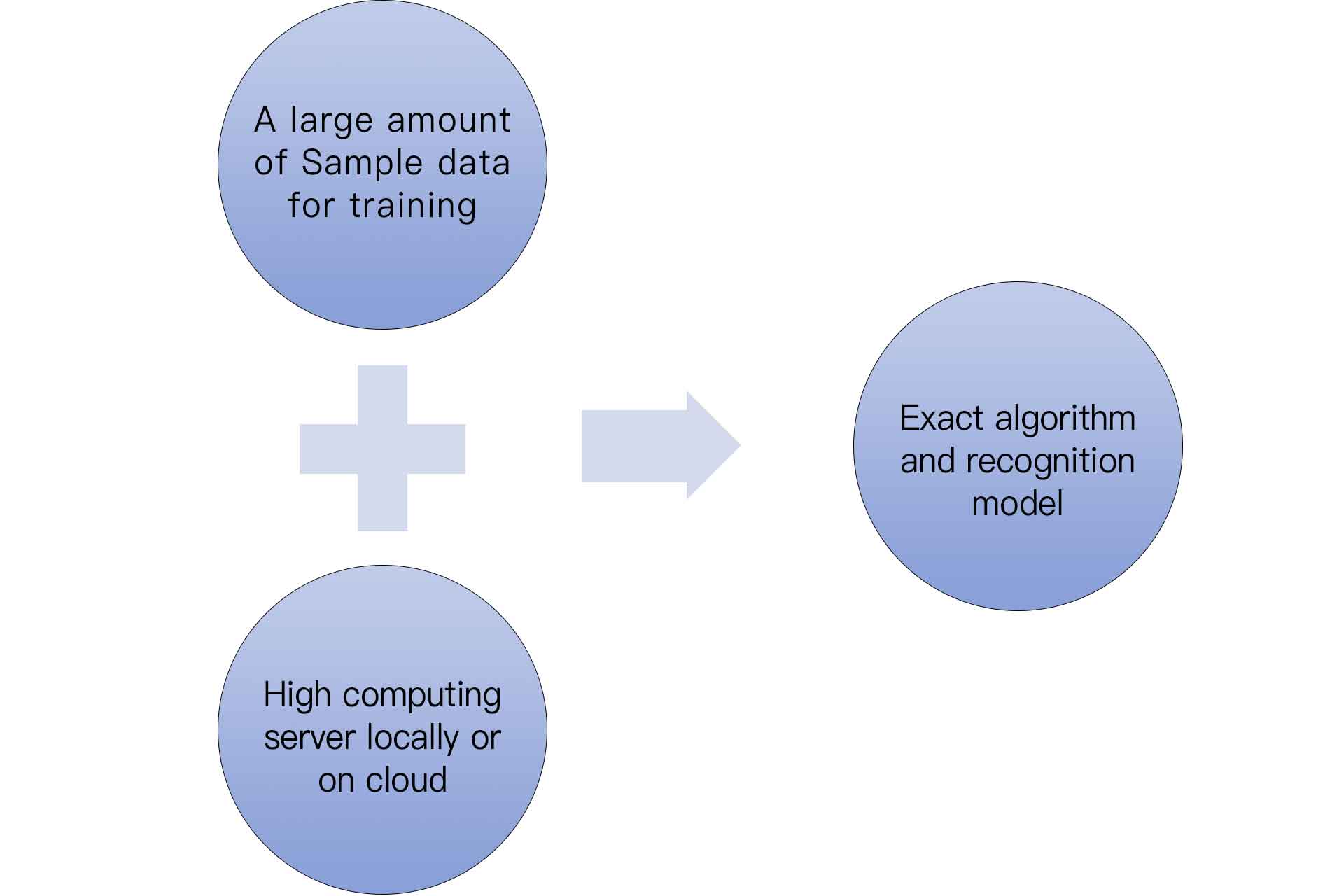
Illustration of Typical Deep Learning Model

Illustration of TD patented ‘DeepCount’
An Innovative Deep Learning Technology-“DeepCount”
TD patented “DeepCount” effectively overcomes the limitations of basic level deep learning through an overall utilization of edge computing, neural network modeling and transfer learning.
Edge computing implements edge intelligence on the edge of the network which is very close to the end user or data source. It is based on this feature that edge computing can achieve high-frequency data exchange and real-time transmission without the need to upload data to the cloud for computing.
The advent of lightweight neural networks has enabled deep learning to improve computational efficiency several dozen times while maintaining similar performance as convolution neural network, which is the core component of modern visual AI system.
The performance of deep learning appears to be unsatisfactory when there is a big difference between the training sample scenario and the application scenario. Transfer learning aims to migrate trained model parameters to a new model to aid in new model training considering most of the data or tasks in people counting are relevant, and therefore the new model can be quickly built up with optimized learning efficiency.
Embedded Intelligent Platform
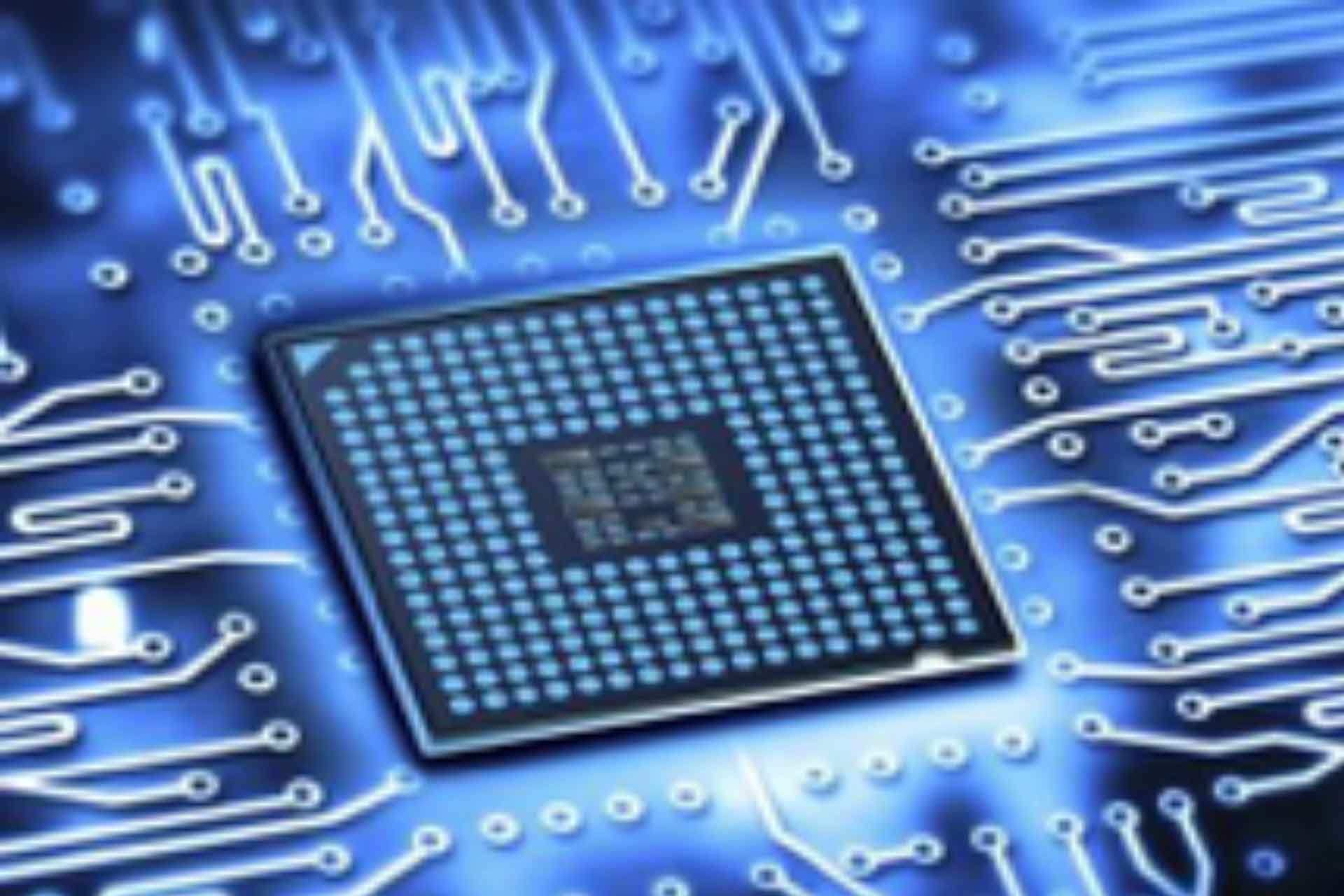